We live in a time which looks to be the shaping of the next industrial revolution.
Technology and AI are developing at neck-breaking speed and the ways people and organizations work are now changing on, quite literally, a monthly basis.
The latest game-changing thing is Generative AI - you have most likely heard about it in the ChatGPT form, although the latter is just one example of GenAI applications.
Given everyone is going mad about it - we have decided to share our thoughts of how this new technology can be used to analyze customer feedback and find insights.
This is not a post about how this technology works but rather a summary of how you may come to benefit from it as well as the risks and limitations to be aware of.
Generative AI represents the cutting edge in artificial intelligence, shifting the paradigm from mere data interpretation to creating new, original content based on learned patterns. It employs sophisticated neural network architectures like Large Language Models (LLMs) to understand the underlying patterns and structures in the data it's been trained on, enabling it to produce original outputs when prompted.
GenAI can mine and synthesize feedback at an unprecedented scale for customer insight, offering a nuanced understanding of consumer behavior.
Generative AI isn't only a promise of improved insights and happier customers.
It's about understanding when its limits get in the way of understanding what your customers really want.
When you trust AI blindly, your revenue streams can take a real hit.
This newsletter is a search for the right balance between those two extremes.
When can we trust AI and when should we look beyond its promises?
So what can this tech do for you in your quest to better serve your customers?
Below are just some of the successful applications I am seeing today.
Narrating charts and data tables
The age-old task of explaining in a few short sentences what is shown on a chart, be it a bar chart comparing segments or a line chart with NPS over time, is getting automated.
Generative AI has proven to be very capable of narrating the main story that is shown on a chart, be it a particular segment standing out or a sharp drop in NPS.
The implication of this is that analytics is about to become a lot more accessible to audiences outside of the data analytics and BI functions.
This in turn will enable companies to finally get to the democratization of information that we've been hearing about over the last decade.
We know that doing CX well, in a way that truly impacts business growth and enables profitability can only be achieved if everyone in the organization has access to the same data points.
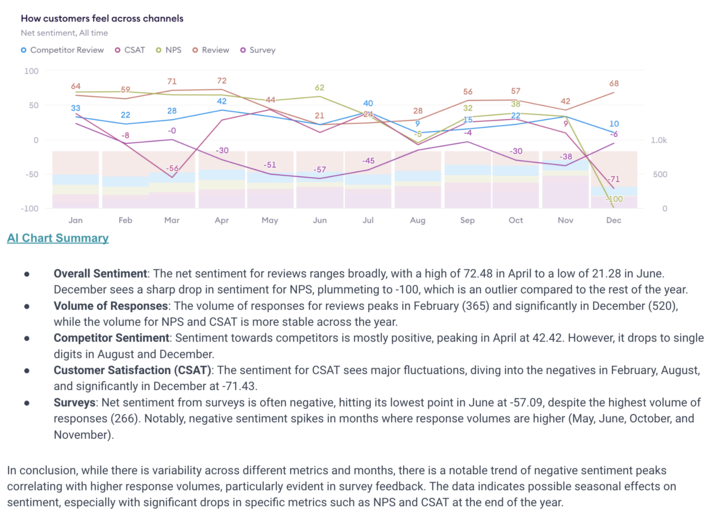
This capability becomes supercharged though when paired with the next application of generative AI.
Writes summaries of customer feedback in real-time
Personally, this is where I’ve spent countless hours and sleepless nights - going through hundreds if not thousands of customer comments to figure out exactly what the customers’ issues as well as what parts of the product or service they love.
This is where GenAI can add the most value right now - reading and summarizing large volumes of verbatim comments to pull out the most important insights.
It is important to couple summarization with a robust data pre-processing pipeline to avoid GenAI hallucinating, which it really likes to do; more on that later, though.
When it works properly it is truly game changing - it is almost like having a sidekick analyst who can do all the trolling through comments for you! A co-pilot, if you will.
Check out some of the examples of summarisation below.
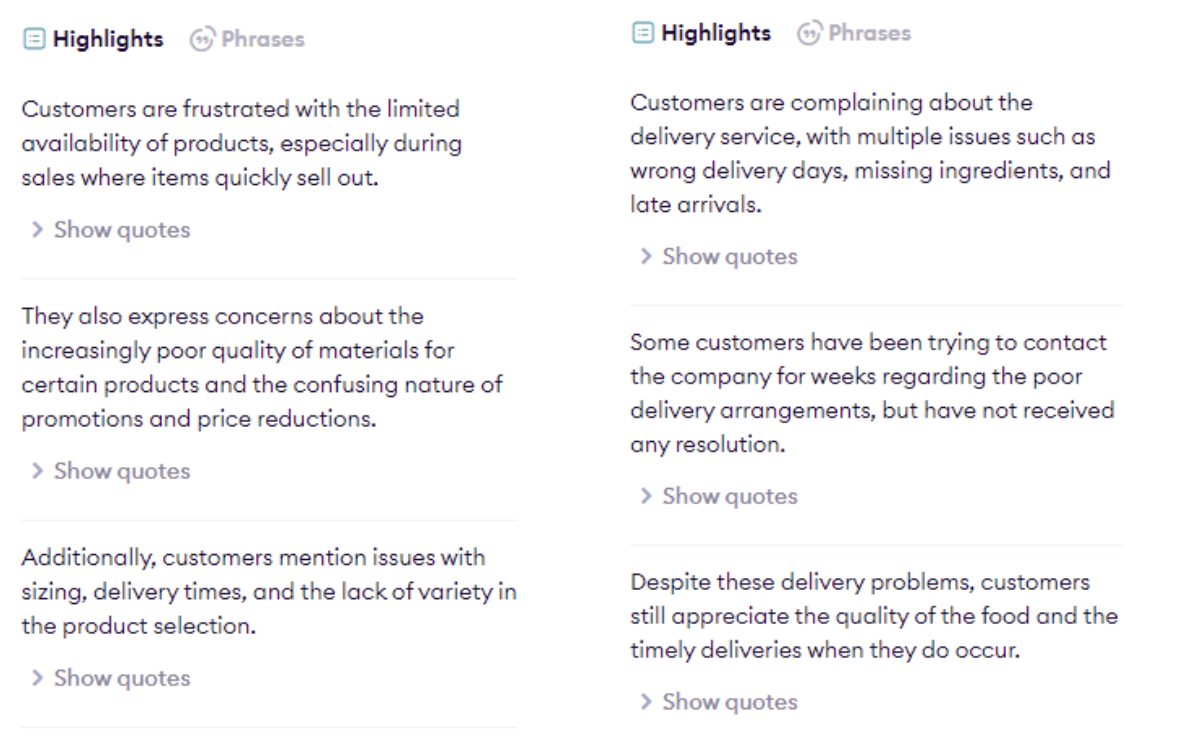
Risks and Limitations of GenAI
If there is one thing we’ve learned about GenAI at Chattermill is that it looks wonderful until you check the results.
That’s where the wheels come off.
We found that without rigorous pre-processing of the data through other machine learning algorithms coupled with intricate prompt engineering, GenAI outputs are at best amusing “fake news” type of responses and dangerously misleading in the rest of cases.
As we started integrating GenAI into our stack we were shocked at just how much it hallucinated which included citing things that just were not true about the data it was analysing and giving wildly inconsistent answers to the same question.
The reason behind this is GenAI models are training to give what looks like credible answers but there is very little computational intelligence in terms of data analysis and validation there.
We were only able to curtail these hallucinations and get the GenAI summaries to be correct and reliable from a quantitative perspective only after we integrated formidable preprocessing, post processing and prompt engineering.
Pre-processing involves feeding the GenAI analytically robust quantitative aggregations, allowing the system to focus on generating qualitative insights, thereby minimizing its engagement in less reliable quantitative analysis.
Post-processing then serves as a quality control barrier, employing safeguards and validations to intercept and rectify errors before dissemination.
Concurrently, refined prompt engineering plays a critical role; meticulously designed prompts boost the AI's comprehension, steering it toward precise, reliable outputs by clarifying the required context and depth of information.
This triad of strategies synergistically fortifies the GenAI against inaccuracies, enhancing its dependability.
Looking to the future
Regardless of the current limitations and the overreaching “creativity” of current GenAI models, the future for this technology is looking very bright!
I feel that it will not be too long before a well tuned GenAI model will be able to play the role of a fully fledged insight analyst that will be able to not only analyse the data but also suggests improvements to data collection and survey design, proactively inform of suspicious trends as well as make relevant recommendations on what to do with the insights.
Will this technology replace some insight analyst roles - yes, to be absolutely realistic, I think it probably will.
However, the good news for anyone worried about their future job is that this is an opportunity to move away from hands-on data analysis into functional teams such as Product where Data + Human judgment and communication skills will be crucial for everything from agreeing on priorities to innovation and new product development.
Ultimately however, it is the customers who will benefit the most from this technology as their voice will be much easier to “hear” through the organization.
Instead of their response being obfuscated behind a bar chart, GenAI can really bring their feedback to life through summaries and suggestions.
The way I see it - GenAI will help bring the customer closer to the company and the people who build the products and services.