Generative Artificial Intelligence (AI) is transforming business before our eyes, but not everyone is rushing to embrace it. Understandably, many people are concerned about its suitability in an enterprise environment or are skeptical it can provide true value.
Yet according to Forrester’s Predictions 2024: Exploration Generates Progress report, 60% of skeptics will use (and love) generative AI whether they know they’re using it or not. Chances are you’re using Gen AI every day and have been for some time. It’s embedded in tools from email and social media to search engines and customer service chatbots. If you’ve taken a suggestion for wording or an offer to rewrite text, you’ve probably used Gen AI.
Granted, the early hype hasn’t been helpful. The news has been littered with Gen AI party tricks, but do we really need a peanut butter sandwich recipe written in the style of William Shakespeare? Is there commercial merit in animating scenes from Breaking Bad with a Hayao Miyazaki flair? No. No there’s not.
As someone who has worked with neural networks since 2005, I’ve seen firsthand the immense potential of Gen AI and understand the apprehension from customers contemplating using it. With the right approach, Gen AI can provide strategic insights that are invaluable to how you run your business.
In this article, I’ll explore the barriers to adoption we’ve seen and how Lyra AI, Chattermill’s proprietary AI architecture, which includes Gen AI, overcomes them.
The why behind Chattermill and Lyra
Chattermill is a software company. As the VP of Data Science, I have a technical role – there’s nothing surprising about that. But what most people don’t realize about Chattermill is tech is not our primary focus. What motivates us most has always been solving a problem using technology – not creating the technology first and then finding ways to apply it.
It’s such an important nuance for people thinking about investing in AI. I’ve been in market research my entire career – so has Dmitry Isupov, one of Chattermill’s co-founders. My experience is in market research, data science, and big data analytics both as a software provider and as a client. In a previous job, I was Head of Research Analytics at Sky. My whole role was to understand customer data to improve Sky’s offering.
AI and the “So what?” conundrum
Chattermill developed Lyra relying on our firsthand experience of working with customer feedback data and using it to make business decisions. That’s why the “So what?” question is really, really important to me. I’ve been in that frustrating situation of not having a good way to easily get the answers I needed even though I had all the data in front of me.
Understanding customer feedback at scale
I do love AI but that’s not why I come to work every day. Everything we do is to address an actual pain point, which is to understand customer feedback at scale. That’s the genesis of Chattermill and the guiding light for Lyra development.
What I realized when I was working at Sky was that the kinds of questions I asked of the data were very different than people in my team who were responsible for specific areas. They wanted to know what programming was most popular with young viewers on weekends between 5:00pm and 6:00pm in northern England, for example. I wanted to know how the brand was performing against competitors like Virgin. It was all in the data and we both needed to consider large volumes of data to get the right answer.
That experience informed development for Lyra. We wanted to focus on all the ways customer feedback is useful in an organization. Lyra was developed so it has broad use for anyone in the business who could benefit from customer feedback data.
But it isn’t only about servicing the immediate needs of different roles in an organization. We were also interested in developing a product that was a lot more strategic for the business. Our clients are looking for information on high-level trends in customer feedback data. They want to know what’s changing in their data over months, if not quarters or years. We want to help them track what people are thinking and feeling over time and understand why that’s important for their business so they can respond in a strategic way. We want to help them improve their customer experience.
Outperforming rule-based lexicon AI models
Customer feedback can shine a light on operational problems that are hard to quickly identify without the benefit of large volumes of data. What’s causing delivery issues? Has a change in service providers improved or hurt your operation? Is a particular vendor delivering subpar quality? That level of granularity and nitty-gritty detail is invaluable for a product manager, especially in hypercompetitive markets.
Chattermill developed a Natural Language Processing (NLP) platform to deliver these kinds of insights. It’s far superior to rules-based lexicon models that require you to know the words and phrases people are going to use in customer feedback. What if you don’t? Consumer behavior and the language they use changes all the time.
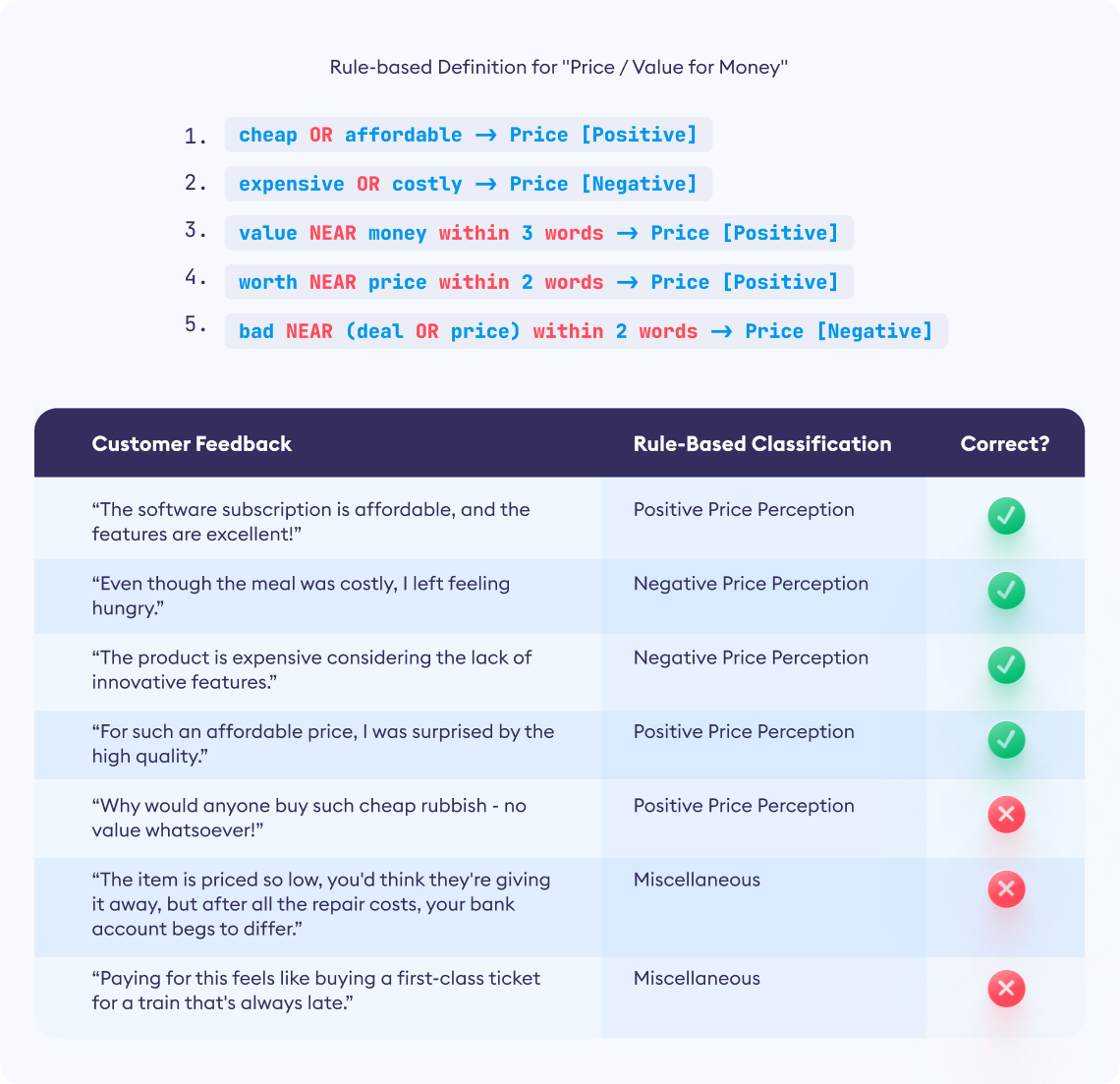
That’s why our open-ended text analysis is so important. A product manager can’t do much with high-level conceptualizations of data. Lyra phrases are things that are expressed in the voice of the customer. You can look at a theme, and then you drill down into the nuances of what customers are actually saying. For example, a customer might have a theme for product returns. If you’re seeing a spike in returns for a popular item, you can pinpoint the reason. You can discover if it’s because something in the product has changed, the product description is incorrect or confusing, or the product has gone out of fashion with customers for a reason that has nothing to do with your company or your product.
Why ChatGPT isn’t the answer for CX data
ChatGPT is getting a lot of attention because it has a broad application. It is a Large Language Model (LLM) and people can use normal language when using it. This natural language interface (NLI), a vision first expressed in the 1960s as computer science was being formed as a discipline, has also made ChatGPT popular.
However, one problem is ChatGPT is designed for general purpose use and lacks a deep and genuine customer experience (CX) understanding. It’s also been trained to produce results that satisfy human evaluations rather than generating factually correct results. It’s famously inaccurate, frequently generating “hallucinations”, or responses that aren’t present in the data. It’s confidently wrong but that confidence can give a false sense of security that you’re getting valuable insights.
A joint study by Stanford University and UC Berkley from 2023, How Is ChatGPT’s Behavior Changing over Time?, showed answers became increasingly worse when analyzing open-ended text. GPT-4’s response rate dropped from 97.6% in March to 22.1% in June. It also became less willing to offer opinions about the text it was analyzing. Similar degradations in responses were observed in different tasks. The researchers concluded LLMs can change substantially in a relatively short amount of time, highlighting the need for continuous monitoring of LLMs like ChatGPT.
Another major problem with ChatGPT is it is non-deterministic, meaning it does not consistently provide the same answer to the same question. That’s a big problem if you’re using it for business decisions. We’ve designed Lyra to be precise and consistent. You can rely on the insights it provides and know the responses you get won’t change from one day to another, because of the focus on precision and accuracy.
Lastly, ChatGPT isn’t updated that often. It’s impossible to respond to market conditions and your own customer experience if you’re working with a general purpose AI that’s based on old training data.
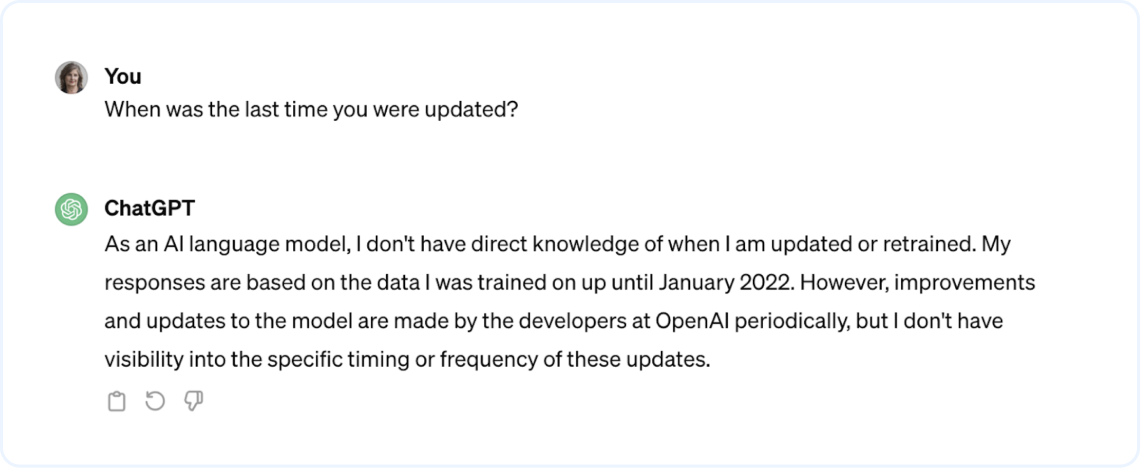
Building trust into Lyra
Lyra is trained on high-quality customer feedback data from various organizations, ensuring that it “learns” from the best subsets of data. The self-supervised learning approach allows Lyra AI to extract patterns across datasets, improving its accuracy and reliability. We’re careful about how we train Lyra and ensure it’s not exposed to inaccurate or overly noisy data. In technical terms, this is commonly referred to as ‘fine-tuning’, and Lyra is based on multiple LLMs fine-tuned for customer-centric data analysis.
Chattermill emphasizes the importance of controllability and the ability for clients to make changes in their own environment. If any mis-tagged comments or labels are identified, Chattermill works directly with clients to address and correct them, which enhances the trustworthiness of the system. Overall, the combination of high-quality training data and the ability for clients to make adjustments ensures that Lyra maintains trustworthiness in its analysis and insights.
Why building your own AI isn’t worth it
It’s hard to think of a use case where it makes sense to build an in-house technology like Chattermill, unless you require incredibly niche expertise. To provide context, Chattermill has an engineering team and a data science team. I’ve been working with neural networks for nearly two decades, which is a long time for AI. When you dedicate a budget to software like Chattermill, you get the benefit of all the experience and investment of the vendor for a fraction of the cost it took to produce the product.
Building a product that works at scale and is reliable, robust, and trustworthy is hard, and it requires a dedicated team of engineers and data scientists. Those skills are in high demand and short supply. The complexity and cost associated with maintaining all the necessary infrastructure and resources are substantial, especially when you consider the massive amounts of data and associated storage required to train an AI system. It’s far more practical and efficient to buy a solution like Chattermill rather than attempting to build it in-house. Even if you can build your own customer feedback analysis tool, maintaining it over time and keeping up with technology changes can be crippling. Trust me; I say this as a former in-house Data Science team leader who understands the challenges faced by internal teams such as shifting priorities. In the case of AI tech, this organizational complexity is further compounded by the continuous evolution of the natural language processing landscape, which demands specialized, ongoing attention that can be challenging for in-house teams to provide.
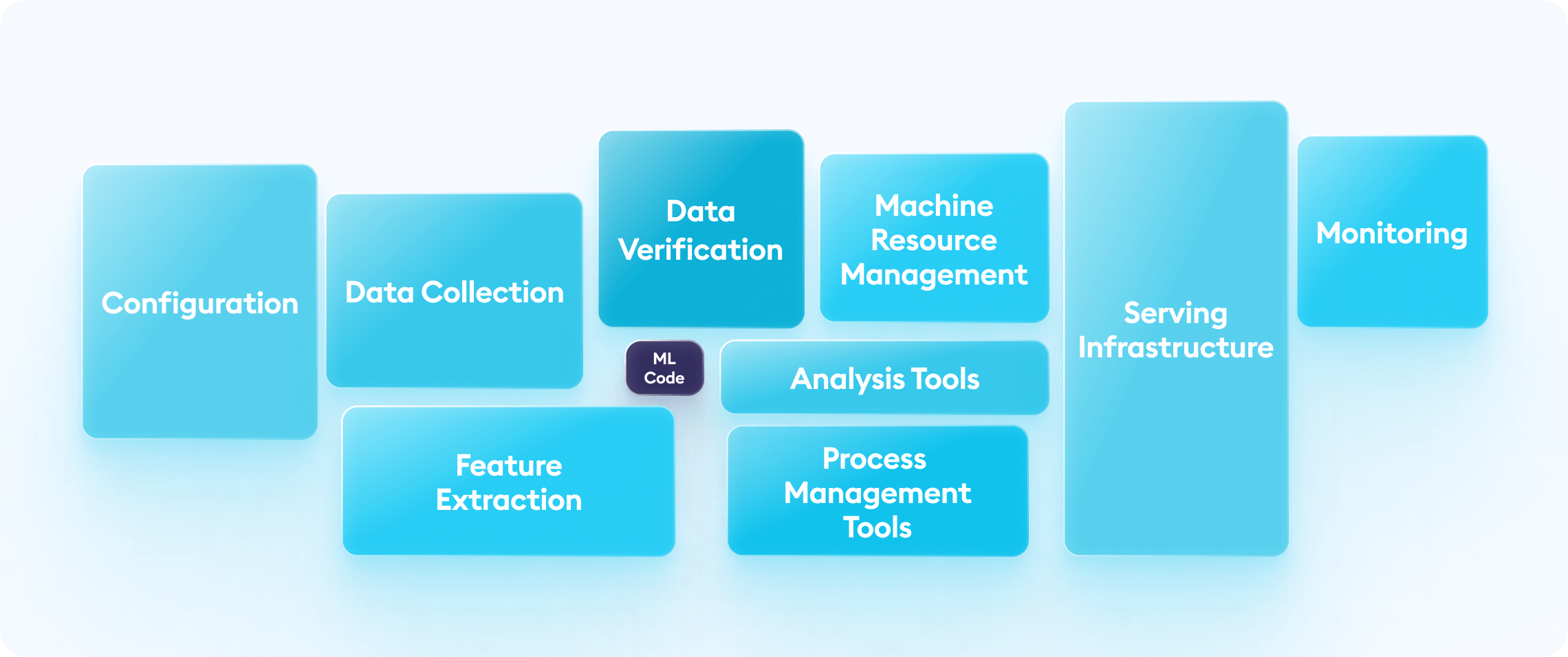
Hidden complexity - the components of an ML production pipeline (Source)