When did you first become aware of artificial intelligence (AI)? Notwithstanding science fiction, when did you realize AI has practical applications for business? For most people, that door opened in late 2022 when ChatGPT was released to the public.
What’s remarkable is how quickly the business community has embraced AI. Aberdeen Strategy & Research reports nearly 80% of companies are turning to AI for their data-driven business activities, specifically customer interactions.
It’s no wonder. Aberdeen also reports using AI across key business activities leads to 81% greater decreases in service costs and more than five times growth in annual company revenue. The top investment is in customer analytics, with 43% of businesses saying it’s where they plan to put their money.
While it’s great that the public is seeing opportunity and practical uses for AI, not all AI tools are ready for commercial use. For those products that are in the market, not all of them have the architecture to support robust, data-driven decision-making.
And, for those that do, none of them are like Chattermill and 100% focused on analyzing customer feedback data. Lyra AI, our proprietary technology, uses a blend of AI text analysis techniques to build your CX Intelligence into a powerhouse decision-making platform.
In this quick guide, we’re breaking down the differences in AI methodologies for text-based analysis to show you why Lyra is really the only choice for companies who want to use deep customer insights for decision-making.
Quick definitions for terms used when describing GenAI
Before we dive in, it’s worth mentioning terms that get thrown around when discussing GenAI.
What is Natural Language Processing?
Natural Language Processing (NLP) is a crucial component of AI that enables computers to understand and work with human language in increasingly sophisticated ways. NLP allows you to enter text as if you’re speaking with a human and receive a reply from a computer in a similar style of language.
What is a Large Language Model?
Large Language Model (LLM) is a category of machine learning models used for NLP tasks. They process and generate human-like text, understand sentiment, answer questions, and perform translation, among other tasks. LLMs have transformed AI technology by mimicking human language and reasoning across industries.
What is supervised and self-supervised learning?
Training an AI model can be performed using two methods – supervised or self-supervised.
- Supervised learning is when the training information provided to an AI technology is controlled by the people who develop or operate it. It relies on manual setup and explicit knowledge about what’s in the data.
- Self-supervised learning automatically learns from the structure of the data. It’s a subset of supervised learning but is more scalable and can expand on supervised learning.
Rules-based lexicon
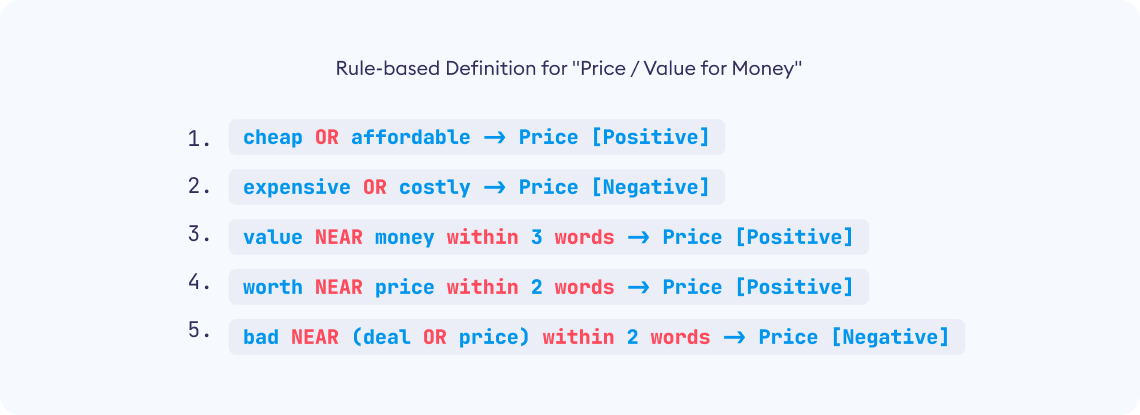
Rules-based lexicons are databases containing words along with predefined rules. Emerging in the 1950s and 1960s, they were essentially dictionaries used by AI to process and understand language and categorize textual data. You read that right – AI was in use for decades before the internet was widely adopted.
As rules-based systems evolved, they became more sophisticated and could understand relationships between words and phrases to determine sentiment.
How rules-based lexicons work
A rules-based lexicon relies on the person training the technology to know or anticipate what kind of words and phrases will be encountered in the text being analyzed. Words and phrases are assigned positive and negative sentiments. Text is scanned and the rules-based lexicon determines which words are negative and which are positive. It calculates an overall sentiment rating based on the percentage of positive and negative words in the text. Typically a rules-based lexicon will deliver a “positive” or “negative” sentiment rating, but some will also include a “neutral” rating.
- Pros: Simple and consistent approach to topic and sentiment analysis.
- Cons: A lot of manual input is required to set up and maintain rules with supervision required from trained linguists, analysts, or engineers. Aligning the results with business process is laborious. A rules-based lexicon is a pioneering form of text analysis that does not support nuanced expressions and can return false positive and false negative ratings.
- Maintaining at scale: Poor
Overall suitability of rules-based lexicon for customer feedback analysis
Rules-based systems are extremely limited in capturing the subtleties and nuance of language, including slang and colloquialisms. They require substantial resources and an ongoing commitment to implement and maintain them effectively. While they are known for consistency, a rules-based lexicon is a simple approach to text analysis and often needs to be supplemented with more advanced AI methodologies to develop true CX Intelligence.
Thematic Analysis and Neural Embeddings
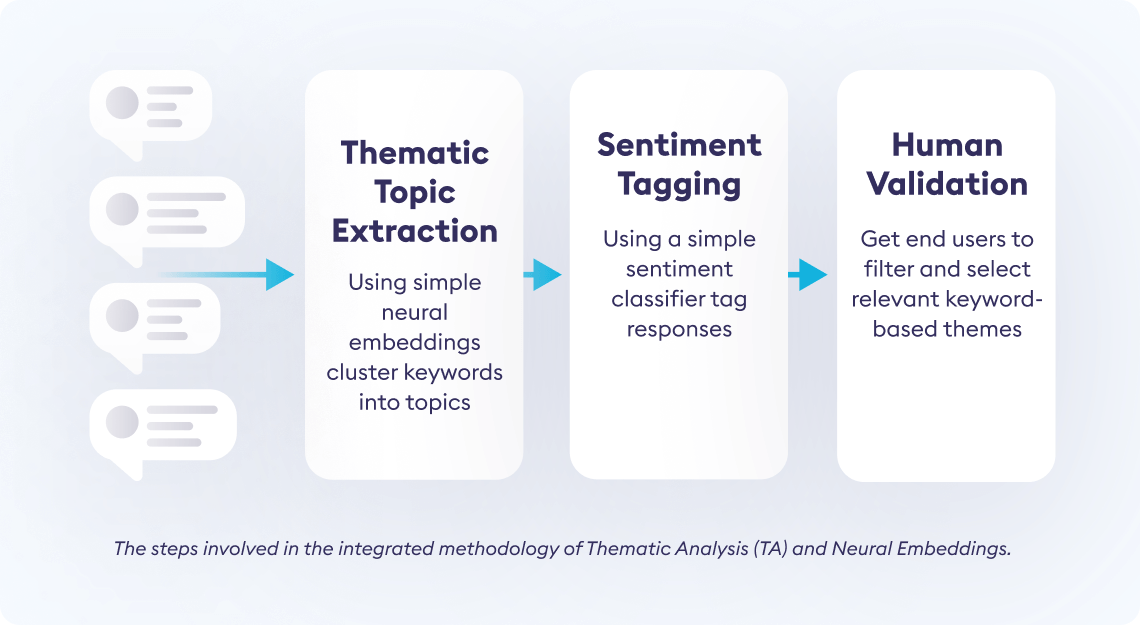
Rooted in Good Old-Fashioned Artificial Intelligence (GOFAI), thematic analysis (TA) gained attention in the 1960s and 1970s. This method focused on identifying and interpreting emerging themes or patterns in data. Early use cases were for psychology, sociology, and anthropology.
Neural embeddings are a technique used to represent words, phrases, or concepts as vectors in a high-dimensional space. They allow for the analysis of relationships and similarities between different words or concepts. Neural embeddings were first introduced in the early 2000s in a neural language model.
How TA and neural embeddings work
TA requires data coding to identify and tag themes occurring in text-based data, then categorizes them into positive or negative sentiments.
- Pros: TA provides a dynamic approach to text analysis, allowing you to create themes on the fly. Themes and patterns surface organically from the data, which preserves authenticity.
- Cons: Heavily reliant on human interpretation, TA requires manual data inspection and the validation of key phrases for each theme. Personal bias easily creeps in as a result. They are rudimentary compared to modern NLP techniques.
- Maintaining at scale: Poor
Overall suitability of TA and neural embeddings for customer feedback analysis
TA continues to evolve and recent integrations with LLM-based neural embeddings mitigate some limitations. Despite the advances, this blended method is no comparison for the performance of supervised, fine-tuned LLMs for topic and sentiment categorization, making them less than optimal for CX Intelligence.
Large Language Models
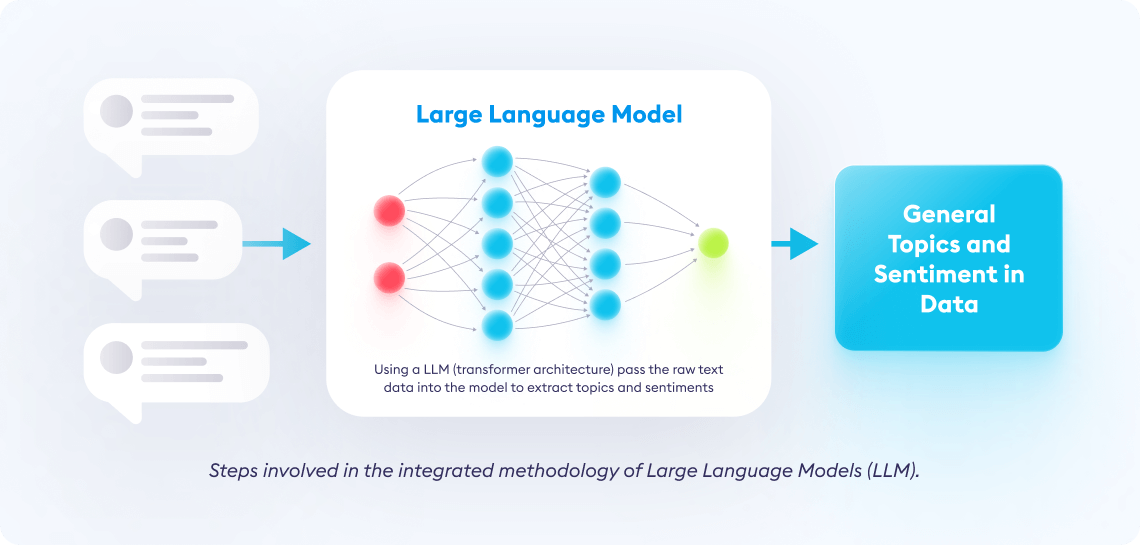
LLMs originated in the study of neuroscience and artificial neural networks. Architecture for modern LLMs dates back to the mid-2010s but only became widely known relatively recently when models like OpenAI’s GPT-3 were introduced in 2020.
How LLMs work
LLMs are trained on massive amounts of text data – often containing billions of words – to learn complex patterns and connections between words and phrases. They capitalize on deep learning principles to generate human-like text. Despite their sophisticated design and capabilities – not to mention the global hype surrounding them – LLMs have drawbacks when it comes to analyzing textual data like customer feedback.
- Pros: LLMs can create text responses so similar to human communication it can be impossible to determine whether it’s man or machine. The more they’re trained, the more human-like they become.
- Cons: LLMs can be wildly unreliable, even comical in delivering incorrect answers. These “hallucinations” may be entertaining but they’re also a sign of unreliability. LLMs are not adapted to specific tasks or specific industries.. Insights may be incorrect due to a lack of context or domain knowledge.
- Maintaining at scale: Excellent
Overall suitability of LLMs for customer feedback analysis
LLMs hold promise, especially if they’re carefully trained with high-quality data. Closed LLMs provide better quality assurance over open systems where training is not regulated or monitored. Even though human-like answers can provide a false sense of security, they are not an ideal choice for precise and accurate CX Intelligence.
Lyra
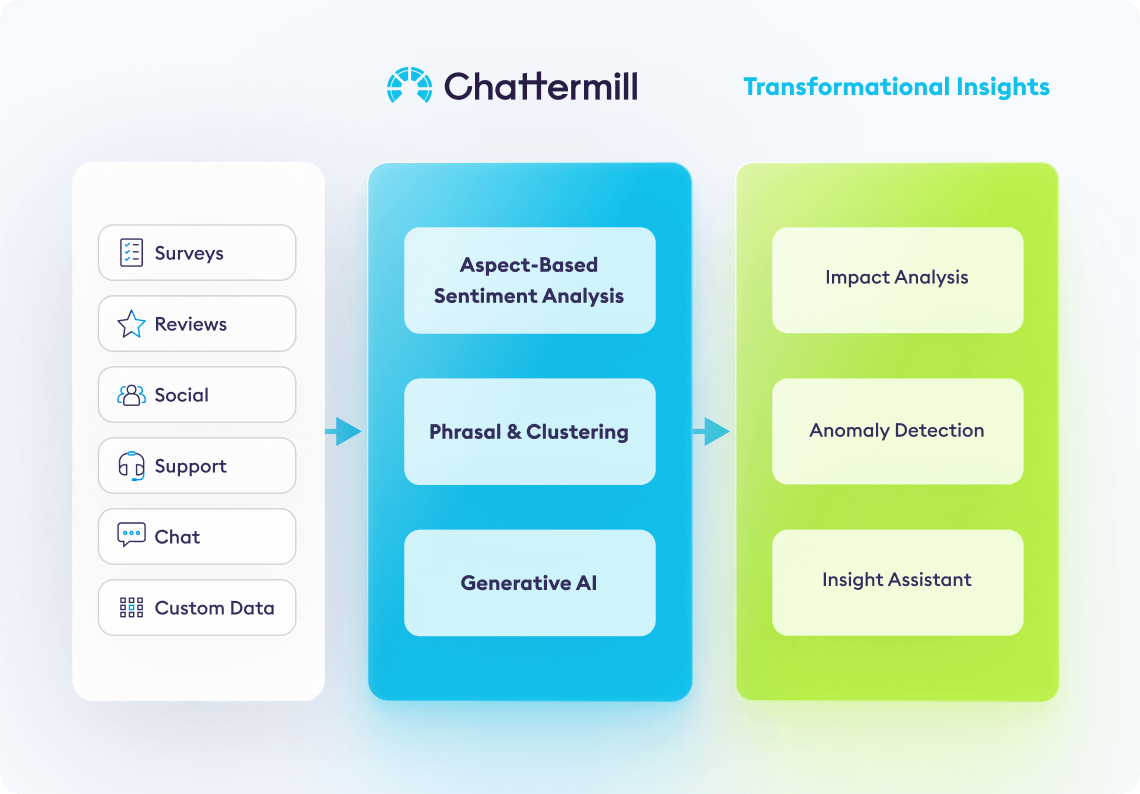
Lyra is Chattermill’s in-house NLP platform used for text analysis. It’s an advanced AI system known for high accuracy and precision when analyzing customer feedback.
How Lyra works
Lyra uses a hybrid of AI methodologies developed from more than a decade of experience working with customer-centric businesses. It combines customer feedback from multiple sources into a single source of truth known as CX Intelligence. Lyra’s proprietary architecture builds on the strengths of each AI methodology to produce transformational insights that drive strategic and operational decisions.
- Pros: Lyra AI surfaces nuanced sentiment analysis from a variety of data sources like email, chat, customer feedback and social media. It provides a high level of customization so businesses can align analysis with business goals and industry nuances. Human-like language powers an Insight Assistant to maximize value and ease of use.
- Cons: Lyra AI relies on the quality of training data, much like any other AI system. Poorly structured or incomplete data can lead to less meaningful insights.
- Maintaining at scale: Excellent
Overall suitability of LLMs for customer feedback analysis
Chattermill’s Lyra offers a unique approach to developing actionable, accurate, and contextually rich insights from customer feedback. The result is CX Intelligence that shows brands precisely what their customers are thinking and feeling, at scale, so they can make data-driven decisions with confidence in near real time.
Why Lyra for text-based analysis?
AI is becoming integral to business success, but not all AI methodologies are created equal. Only one stands out for precision, accuracy, and the contextual understanding necessary for effective customer feedback analysis. Chattermill’s Lyra leverages a blend of AI methodologies to deliver actionable, industry specific CX Intelligence. With Lyra, businesses can confidently make data-driven decisions, enhance customer experiences, and drive Experience-Led Growth. In an era where AI is no longer science fiction, Lyra AI delivers present-day competitive advantage.