When I speak to businesses about tools for Customer Experience (CX), I am often asked, “What is the one approach that works best?” or “Which AI method is the top one for CX?” But I don’t have a single top AI approach - there are lots of AI approaches that work for CX. The trick is to use them all together to build one successful platform that analyzes feedback at both strategic and tactical levels.
There’s no denying that with the arrival of ChatGPT in November 2022, the world has taken a huge leap forward in Natural Language Processing (NLP). Unfortunately, this has opened the door to unrealistic expectations that leave companies with failed projects and poor-fit solutions. Now businesses are increasingly - and rightly - at the peak of excitement, but also at their peak of skepticism. This is especially true since there has been no objective framework for evaluating the multiple disparate technologies under the AI umbrella for their specific uses in CX.
As someone who has worked with AI since 2009, I’ve seen firsthand the difficulties companies face when adopting technology at the peak of impossible expectations - and there’s no sign of this slowing down. In fact, Gartner predicts that by 2025, 80% of customer service and support organizations will be applying generative AI technology in some form to improve agent productivity and customer experience (CX).
The problem with most existing software is two-fold. First, it acts as a tool (think of a single item in a toolbox), rather than as a platform that connects the core strategic and tactical objectives that enterprises need to drive business goals with CX. Second, many providers sell their solution as a silver bullet without acknowledging the enterprise journey required for long-term profitability. The result is often a collection of unrelated tools that are procured at the team level to solve tactical issues, rather than answering strategic pains.
In today's CX landscape, linking tactical issues to strategic pillars is essential for success. The strategic-tactical continuum allows businesses to connect granular, actionable feedback with their overarching customer experience goals. By understanding how tactical issues contribute to strategic outcomes, companies have a continuum that shows that way to connect daily improvements with broader business objectives.
I believe that the prevalence of the disconnection between strategy and tactics in CX is exacerbated in large measure by the difficulty of AI models that rely on keyword-based approaches. For a detailed comparison of different methodologies, take a look at our article, Why Lyra AI Comes Out on Top When Comparing AI Platforms for Text Analysis. The problems created by other models, are the very issues that Chattermill solves by using the right combination of Large Language Models (LLMs) and generative AI to cover the entire strategic-to-tactical continuum.
In this article, I’ll explore the strategic-to-tactical continuum, why it’s particularly important in CX, and how Lyra AI is built to bridge the entire continuum.
The why behind the strategic-tactical continuum and Lyra
First, some background on Chattermill. As Chief Revenue Officer (CRO), I oversee the strategic alignment of our customer success, partnerships, and sales functions, ensuring that we prioritize the customer experience of the businesses that work with us. What people often overlook about Chattermill is that what motivates us is our client’s success and their customer experience - not riding the wave but using AI in a deliberate way to enhance our technology and add deeper insights.
More than any other point in my 20-year career, I’m talking to customers who want to develop a deep knowledge of our technology before they buy. When I was Vice President at Crimson Hexagon, a consumer insights company, initial customer discussions were mostly focused on the connection between strategy and tactics. For example, we had the privilege to work with NIKE Western Europe to prove that large-scale analysis of unsolicited feedback from social media – combined with internal or enterprise sales data – could be a stronger predictor of future sales success and stock production than past reseller figures. The results highlighted the importance of using AI to manage such industrial-scale tasks without losing human-level contextual understanding that would otherwise have severely skewed results. However, during these discussions, I rarely ever saw such a prevalence of skepticism about the effectiveness of technology as exists today.
I believe that customers can't afford to trust the high-level promises of CX tools. And, according to PwC’s 2024 Trust Survey, this is part of a much wider trend towards consumer mistrust. In fact, 90% of business executives think customers highly trust their companies while only 30% of consumers actually do. That gap of 60 percentage points is greater than the 57 points PwC saw in both 2023 and 2022.
I’ve been working in AI my entire career, helping enterprises find the right platform to support multiple use cases so that they can connect to what their customers care about. Now more than ever, I’m endorsing the healthy skepticism buyers have about AI and CX.
What is the strategic-to-tactical continuum?
So, one way I think about this is within Gregory Bateson’s claim that “Without context, words and actions have no meaning at all.” This connection between context and meaning is about the nuances and richness of language, but it’s also about a continuum from no context to deep understanding across many contexts. A good example is the customer check-in experience. Initially, it may appear that a customer is complaining that they’ve had to wait a long time for a flight check-in. When they add that they arrived at peak time, the context changes. Now we can see the expectation of the customer experience changes from a complaint to a qualified statement.
Once the context is established, it’s possible to connect tactical issues like delayed check-ins to strategic themes of speed and convenience. AI that bridges the strategic-tactical continuum can prioritize fixes that align with larger goals, helping organizations to act in ways that both resolve immediate challenges and further overarching CX objectives.
Why precision, accuracy, and recall matter for strategy and tactics CX
What I realized when working at Crimson Hexagon was that different approaches to technology had specific impacts on a company’s ability to analyze feedback for strategy and tactics.
The diagram below shows how strategy and tactics differ along three dimensions that are essential to successful CX:
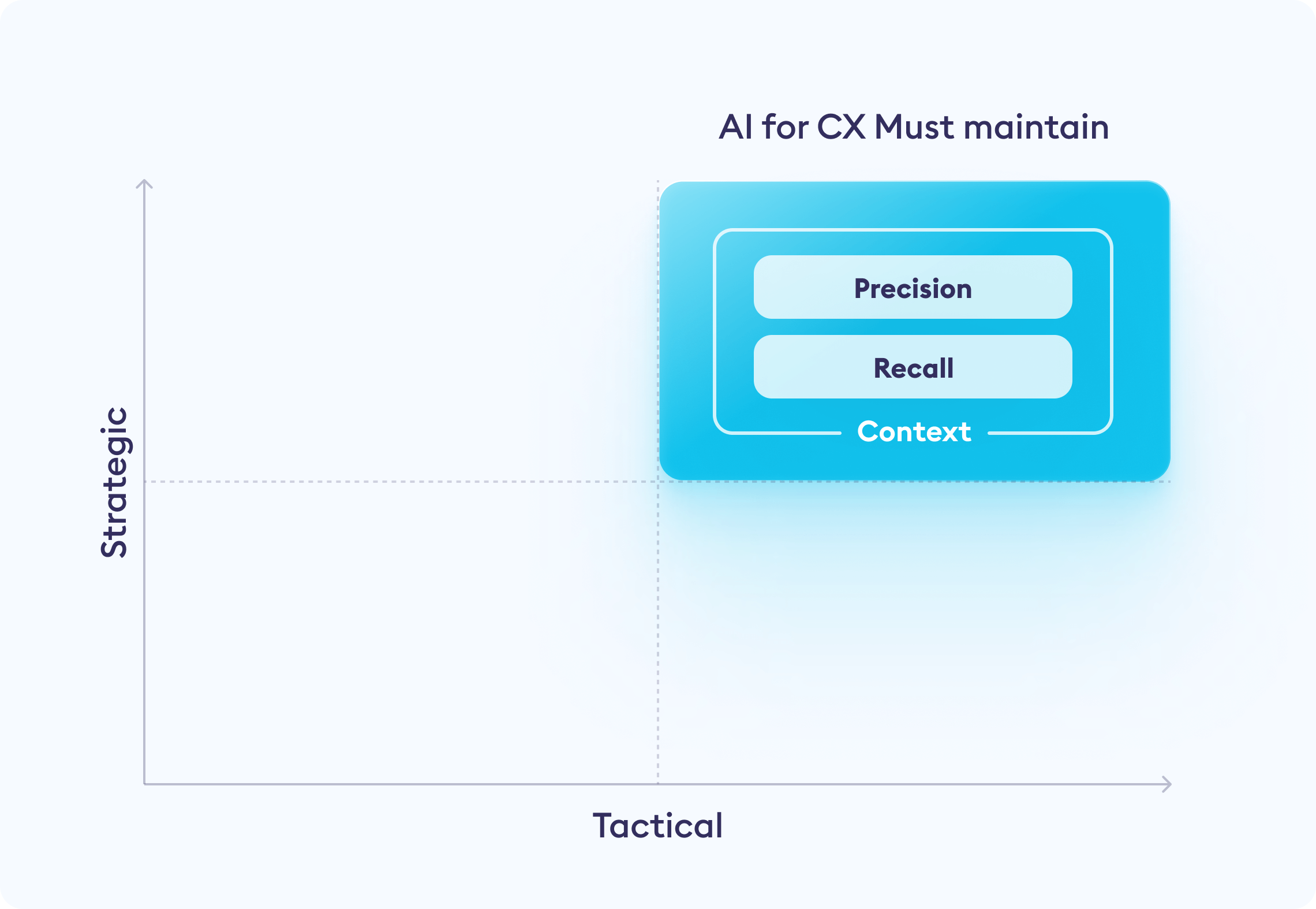
Precision measures the model’s ability to detect relevant elements and differentiate them from other (irrelevant) elements.
- Tactical Level: At the tactical level, keyword approaches can provide high precision because the terms are specific and well-defined. For instance, "app crash" or "payment failure" can be accurately identified and counted.
- Strategic Level: As the analysis becomes more strategic, precision decreases because broader concepts are harder to capture with specific keywords. For instance, "customer satisfaction" or "loyalty" cannot be encapsulated with a single keyword or phrase, leading to under-reporting or over-simplification.
Recall is the measure of how many relevant elements were detected.
- Tactical Level: Keyword approaches often achieve good recall at the tactical level by identifying a significant proportion of direct feedback related to a specific issue. For example, terms like "refund" or "delivery delay" are straightforward to match.
- Strategic Level: At the strategic level, recall suffers because broader concepts involve varied terminology and require more abstract thinking. For instance, "brand perception" may involve indirect expressions that differ vastly. There’s a potential for missed data due to variation in customer language.
Context is important in AI because it allows AI models to understand the meaning of information in a way that is relevant to the situation, such as detecting irony.
- Tactical Level: Context is less crucial at the tactical level, as specific issues can be addressed even without broader understanding. "Login error" or "incorrect billing" requires immediate fixing, and keywords generally provide enough information.
- Strategic Level: Strategic analysis relies heavily on context because understanding customer sentiment and behavior relies on interpreting indirect signals. Keyword approaches cannot handle context well, especially when the meaning shifts based on other information. For instance, "long wait" could be a simple complaint or a justified statement depending on circumstances like time of day, and staff availability.
In order to support successful CX, your AI model needs to support precision, recall, and accuracy as it shifts from tactical to strategic analysis.
What is the problem with keyword-based approaches?
Let’s examine why keyword-based approaches struggle to maintain precision, recall, and context during the tactical to strategic shift.
- Lack of Context Understanding: Keywords focus on specific terms or phrases, often missing the broader context that might change the meaning. For instance, "broken AC" could suggest a negative experience in a hotel review. However, with added context (such as the review mentioning "the staff immediately fixed it and offered a free upgrade"), the overall sentiment might be positive due to excellent customer service.
- Inflexibility: Rule-based systems rely on predefined patterns that are rigid. They struggle to adapt to new language trends, slang, or industry-specific terminology, making them less effective as customer language evolves.
- Overlapping and Double-Counting: When grouping similar themes, keyword systems often double-count or overlap issues due to the inflexibility of predefined terms. For instance, a theme like "customer service" might overlap with "support," leading to inaccurate analysis.
- Lack of Abstraction: Keywords excel in tactical analysis for clear, specific issues. However, when looking at strategic insights requiring higher-level abstraction, such systems fall short. They can't easily categorize broader trends that don't fit neatly into a set of rules.
- Difficulty Handling Ambiguity: Keywords are literal, which means they struggle to interpret ambiguous or nuanced feedback. Sarcasm, irony, or complex sentiment can't be accurately captured, leading to a skewed understanding.
- CX Scalability: As customer data grows, rule-based approaches become harder to manage. The rules require constant updates to maintain relevance, which becomes impractical at scale.
These limitations demonstrate why keyword or rule-based approaches can't span the entire strategic-tactical continuum effectively. Chattermill’s Lyra AI combines various advanced techniques, such as Aspect-Based Sentiment Analysis (ABSA), clustering, and generative summaries. Together these can provide more comprehensive insights for both tactical actions and strategic planning.
Why CX can’t afford for the strategic-to-tactical continuum to fail
The diagrams below shows specific strategic and tactical issues mapped to the X and Y-axis. For example, at the tactical end, a keyword-based system might excel in identifying specific issues like "payment failure" or "login error." However, as feedback becomes more nuanced or broader, such as discussing overall user experience or long-term product satisfaction, the same system can falter, losing accuracy and context.
Let’s take a closer look at a few industry-specific examples.
Example 1: E-commerce Platform
Tactical Issue: "Cart abandonment due to payment failure."
Strategic Conceptual Theme: "Overall shopping satisfaction and trust in the payment system."
Why It Matters: A keyword-based system can easily identify and count mentions of "payment failure" when customers abandon their shopping carts. However, understanding the broader impact of these failures on overall shopping satisfaction and trust in the platform requires more nuanced analysis. For instance, customers might express frustration not only with the payment system but also with the perceived security and reliability of the entire shopping experience. Advanced AI can link these tactical issues to strategic themes, providing insights that help improve the entire payment process and build customer trust.
E-commerce Platform

Example 2: Hospitality Industry
Tactical Issue: "Room cleanliness issues."
Strategic Conceptual Theme: "Guest satisfaction and overall hospitality experience."
Why It Matters: A keyword-based approach can highlight specific cleanliness complaints, but it might miss the broader impact on guest satisfaction and their overall experience. Advanced AI can connect these cleanliness issues to broader themes like "hospitality," revealing how such issues influence guests' likelihood to return or recommend the hotel. This allows the management to prioritize housekeeping improvements as part of a strategic initiative to enhance guest satisfaction.
Hospitality Industry

Example 3: Financial Services App
Tactical Issue: "App crash during transaction."
Strategic Conceptual Theme: "User trust and financial security."
Why It Matters: While keyword detection can identify complaints about app crashes, it requires advanced AI to understand the broader implications for user trust and perceptions of financial security. Users might express concerns about the safety of their transactions and the reliability of the app in handling their finances. By connecting these tactical issues to strategic themes, the company can address not only the technical problems but also implement measures to reassure customers about the security and reliability of the app.
Financial Services App
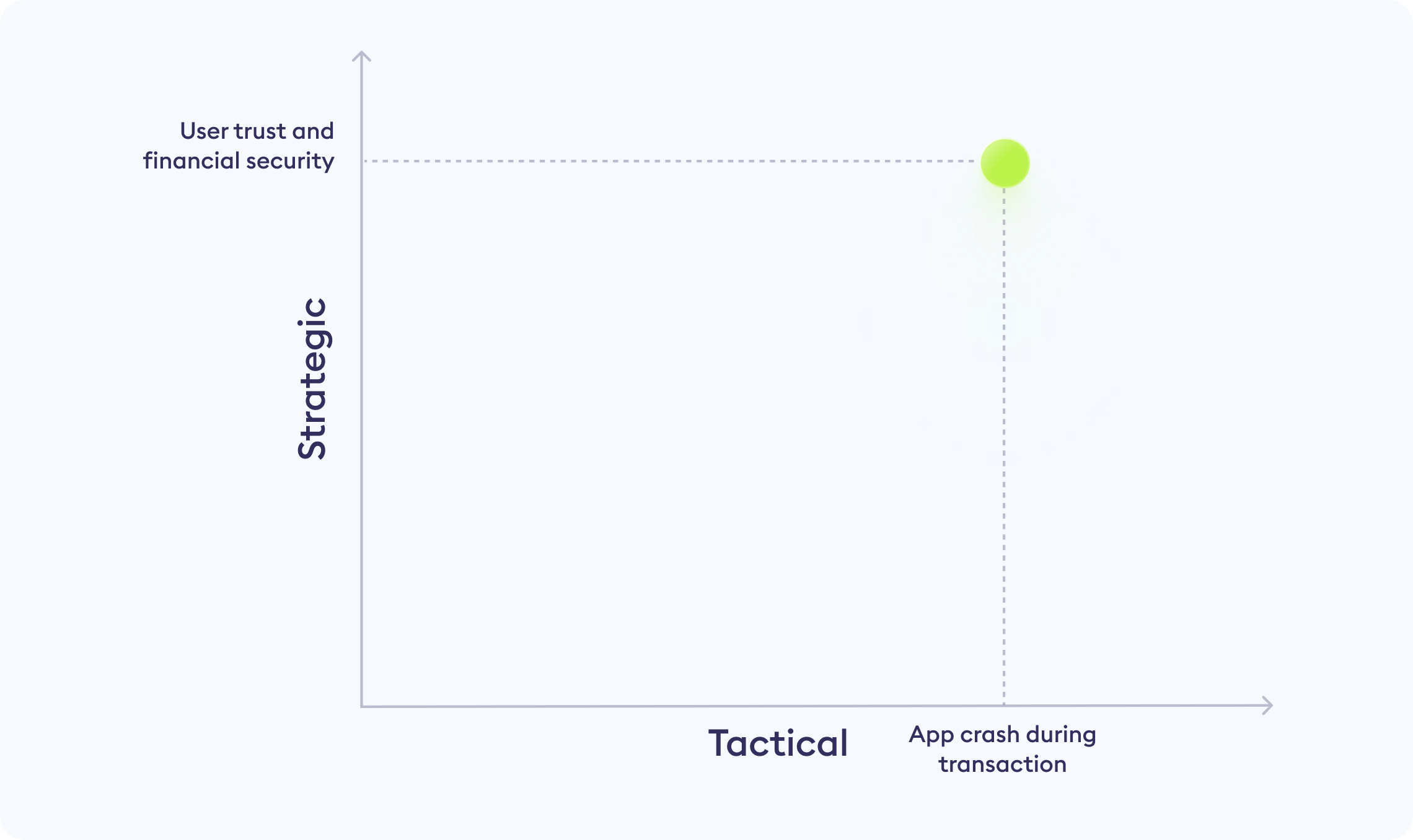
Looking at the Y axis, if I’m using a keyword-based approach, I can maintain precision, recall, and context at the level of granularity. However, if I want to go up the axis to see how different facets of the same experience connect at the business level, I lose that precision and recall. Also, by grouping themes together I risk adding other issues such as overlapping and double counting. For example, your business might monitor “hospitality”, which includes check-in experience, picking up keys, or room cleanliness. Each of those aspects exists on the tactical level but also needs to be rolled up into “hospitality” without losing its context.
Successful customer feedback programs don’t split out strategic and tactical elements - they include both. Our customers are looking for concept-level tracking that isn’t a collection of keywords that have lost their original context. They want to connect strategy and tactics in a continuum for robust business decisions. This is why keyword-based models are so frustrating for me; they can’t give the tactical answers that inform an organization’s CX strategy. Without this, companies can’t come up with new ways of innovating with customer experience that support improved market differentiation.
How Lyra enables the strategic-to-tactical continuum
Chattermill's Lyra AI bridges this continuum by leveraging multiple AI technologies in combination. It seamlessly integrates ABSA, phrasal analysis, clustering, and Generative AI, allowing it to cover the entire spectrum without compromise.
ABSA enables accurate concept-level tracking by breaking down customer feedback into specific aspects, understanding context, and maintaining high precision.
Example: Lyra AI can track broader themes like "customer service" by understanding various aspects such as response time, agent politeness, and issue resolution effectiveness. This contextual understanding ensures that even smaller themes within a larger concept, such as the friendliness of staff or the efficiency of handling returns, are captured accurately.
Phrasal analysis and clustering provide enhanced granularity so that you can track broader patterns, detect emerging trends, and avoid overlapping themes or double counting.
Example: Phrasal analysis helps group similar phrases, allowing Lyra AI to detect broader trends like "late check-out" even when feedback uses different terms. Clustering ensures that overlapping themes are prevented, maintaining a clear understanding of broader concepts.
Generative AI creates natural language summaries that provide customers with concise reports and proactive alerts. It does not perform the analysis but relies on ABSA's rigorous underpinnings to deliver accurate, easy-to-understand summaries. This helps businesses to quickly understand key insights and respond to customer feedback without the need for the manual review of each comment.
Example: After ABSA has analyzed the feedback and captured nuanced insights, Generative AI creates a concise summary that highlights the key takeaways, allowing companies to grasp the most important points without reading through extensive data.
Conclusion: Lyra AI is built to bridge the gap between strategy and tactics in CX
Chattermill emphasizes the importance of a comprehensive solution that is customizable for your business and covers the entire strategic-to-tactical continuum without compromise. Because it doesn’t rely on specific keywords, it won’t double-count or overlap theme issues. It also updates with new slang words and removes the need for manual checking and analysis.
For example, Lyra AI allows retail companies to identify specific product issues like "shipping delays" with precision while also tracking broader concepts like "customer service quality" or "overall shopping satisfaction." This versatility is crucial for a comprehensive understanding of customer feedback, allowing them to make informed decisions and enhance the overall customer experience.
This way, businesses can ensure high accuracy, precision, and contextual understanding, with a flexible and adaptable platform for customer feedback analysis. Lyra’s unique combination of ABSA, phrasal analysis, clustering, and Generative AI ensures that it can tackle any use case - from the most specific tactical insights to broader strategic themes.