Terms like “text analysis,” “sentiment tracking,” and “natural language processing” are thrown around a lot today, as AI-backed inventions continue to change the way we live. Though these topics may seem too complex for the average person to understand, when you break them down they’re surprisingly accessible. Take a look at the examples below to learn about some of the most common steps and processes machines take to “understand” information, and how text analytics can help your business process valuable information at scale.
What is Text Analytics?
Text analytics is the process of taking written information and transforming it into data points that can be tracked and measured. The goal is to be able to extract quantitative data from unprocessed, qualitative information in order to discover patterns and trends within the text. AI allows this to be done automatically and at a much larger scale, as opposed to having humans sift through a similar amount of data.
Everyday Examples of Textual Analysis in Business
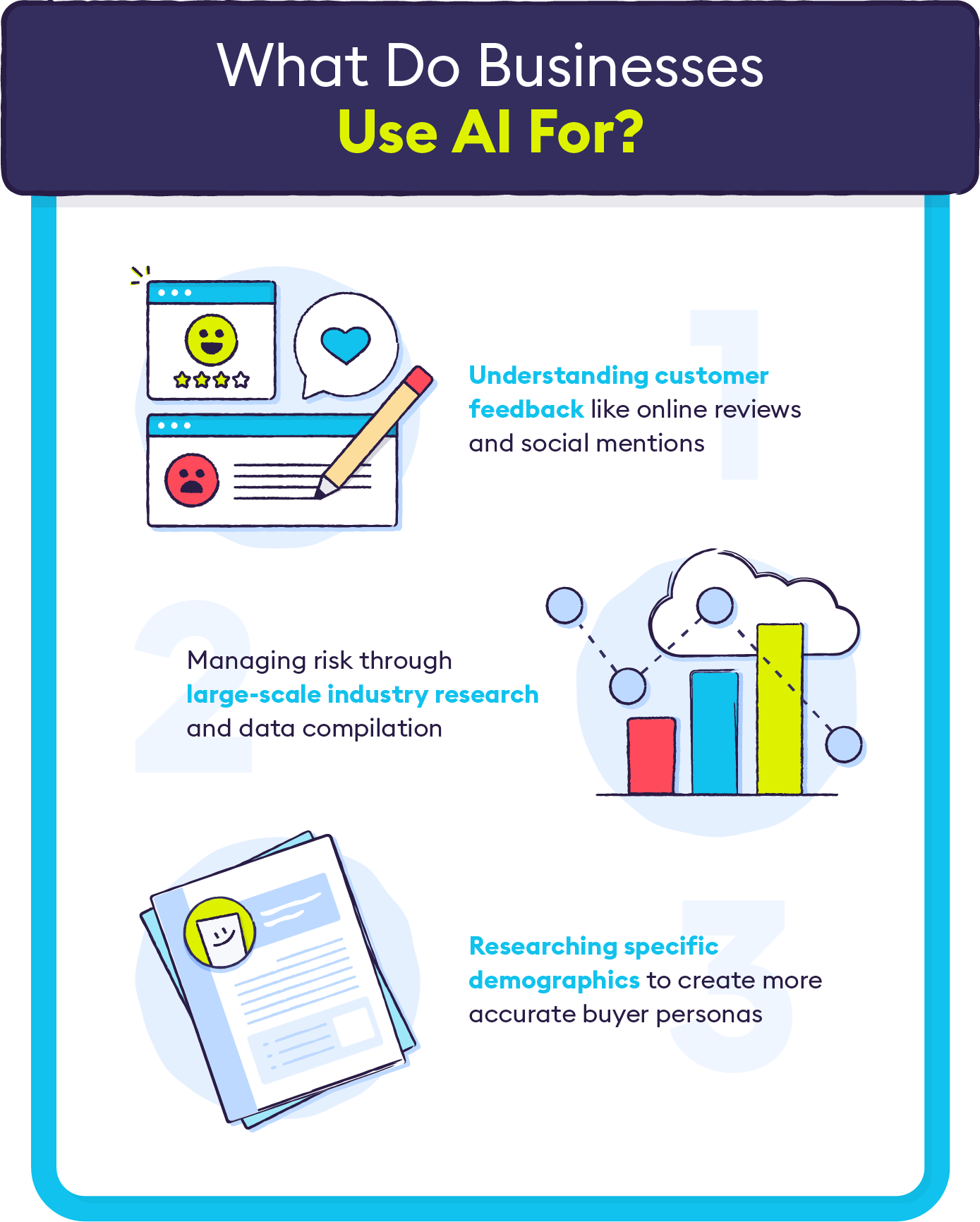
If the definition above sounds a bit vague or confusing to you, here are some real-life examples of how businesses make use of text analytics:
Understanding Customer Feedback: Say a company launches a new product and wants to know how their users feel about it. They can gather online reviews, social media discussions, and feedback from surveys to find out. However, it would take human employees hours if not days to sift through all of this feedback and identify patterns. Instead, AI-backed textual analysis can sort through qualitative feedback to identify patterns and assign quantitative measurements that can be more easily compared.
Why don't you try out our text analytics tool with a customer experience or support survey response?
Targeted Marketing: Text analytics tools can be used to research audience segments, identifying their interests, beliefs, buying and watching habits online, and more. This helps businesses create more accurate and targeted buyer personas, and serve them with ads that are better customized to fit their unique outlooks.
Risk Management: Financial institutions use text analytics to decide whether or not to lend to certain companies. By analyzing qualitative-based information such as news articles, social posts, and analyst reports with AI, banks are able to get a clearer picture of their potential customer and the amount of risk they pose as an investment.
In addition to the three examples above, there are numerous other ways companies use text analysis across industries.
How Textual Analysis Works on a Basic Level

Before we get into the specifics of text analytics functions, it’s important to understand the basic ideas behind how they work. Natural Language Processing (NLP) is a complex topic (after all, it’s modeled after the human brain) but it’s helpful to have an understanding of the process the AI goes through when analyzing a piece of text. There are more steps involved then the ones listed below, but these should be enough to give you an accurate overview.
1. Sentence Tagging and Chunking
First things first, how do machines even recognize language enough to analyze it? The process is basically the same as how we do, though we’re not conscious of it most of the time. The first step is sentence tagging, also known as part of speech (PoS) tagging. The AI bot goes through the text and assigns each word to a part of speech (noun, verb, adjective, etc.). The next step is to break each sentence into chunks, based on where each PoS is. These are usually categorized as noun phrases, verb phrases, and prepositional phrases. Here is an example of chunking: The young woman (noun phrase) is taking a trip (verb phrase) on a boat (prepositional phrase).
2. Parsing
Once the text is tagged and chunked, the AI will do what’s known as parsing. Using the syntax (sentence structure) defined above, sentences can be broken up into different sections or elements, with different traits being pulled in each. This is how more complex text analytics like sentiment tracking works. For example, take the sentence “The young woman is taking a trip on a boat, but her younger brother is jealous.” The bot would be able to break the sentence into two different elements and pull different keywords and sentiments for each.
3. Rule Setting vs Topic Modeling
Now that you understand how AI “reads” text, we can get into how that text is used. AI programs are built to recognize important words, phrases, or other data points within a text. For example, a customer support ticket for an appliance retailer might read “The washing machine was broken when it arrived, I’d like to request a refund.” The AI would pull out keywords like “washing machine,” “broken,” and “refund.”
From here, there are different ways the AI can proceed to use the data. The first, and most commonly-used, is rule setting - what do you want the AI to do once it has the data? You can set rules that text with certain words will be tagged in a specific way, such as being sorted into a category. Though rule setting is more rigorous to set up in the beginning, it is also more accurate since you’re telling the AI exactly what you want it to do.
Another method is topic modeling. Topic modeling is what’s known as unsupervised machine learning, in which a bot categorizes texts based on what it detects as common themes, words, or patterns within each of them. The advantage to topic modeling is that there’s virtually no set up involved, so you can get started on your data analysis very quickly. The disadvantage, as mentioned above, is that this method typically isn’t as accurate as supervised learning. It’s up to you to decide which approach is right for your business and the types of qualitative data you need to analyze. For the examples below, we’ll be assuming that the businesses are using AI with set rules.
5 Text Analytics Uses + Examples
Here are some of the most common ways text analytics tools are used by businesses, along with real-life examples for each.
1. Language Identification
One of the most basic text analysis functions is language identification or language detection. These capabilities are a must for businesses with a global audience, which in the age of online, is the majority of companies. Many text analytics programs are able to instantly identify the language of a review, social post, etc. and categorize it as such. Though this is easy enough for a human to do with widespread languages like English and Mandarin, it becomes much more difficult with closely-related languages such as Norwegian and Swedish, for example.
Real-World Example: A global eCommerce company receives hundreds of online reviews, complaints, and customer service tickets each day. To save money and time for their customer service team, they utilize text analysis with language detection.
When the team receives a piece of qualitative feedback that they need to address, say, in French, the AI automatically identifies the language and forwards it to their French-speaking rep to handle. Otherwise, someone would have to try to manually identify each language for each review and forward it to the correct person each time, which is no small task for a large company.
2. Topic Analysis
Topic analysis is another basic function of text analytics programs. By identifying keywords, an AI bot scans a piece of text and assigns it to a certain topic based on what it pulls as the text’s main theme.
Real-World Example: A local technical college uses AI to analyze reviews of the classes to see what they can improve. A bot using topic analysis would scan through each review and pull out keywords, such as “teacher, course material, schedule, expensive” etc. Based on these keywords, each review would then be assigned a broader topic based on where the keyword fits in. For example, reviews mentioning “night classes” could be categorized in “course availability.” Though this is useful on a basic level, topic analysis becomes much more effective when combined with other methods such as sentiment tracking, to provide more nuanced analysis.
3. Intent Tracking
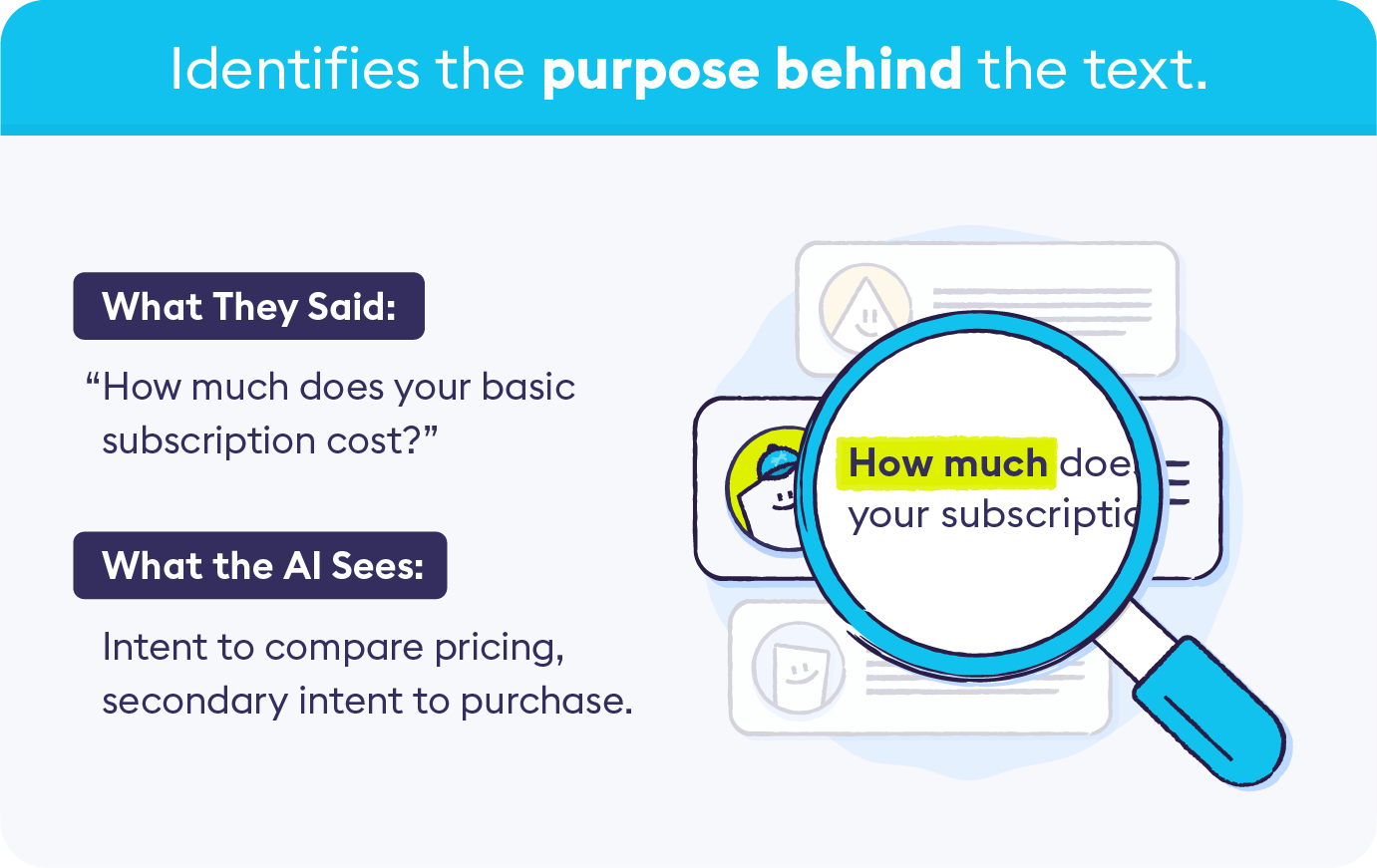
Intent tracking is similar to topic analysis but takes things a step further. In addition to pulling out keywords to identify what a piece of text is about, AI using intent tracking will be able to detect the purpose or intent of the text as well. Basically, what was this person’s intent when writing the text? This is particularly helpful when building contact lists, customer segments, or determining where a piece of communication should be sent when creating a customer service workflow.
Real-World Example: A popular lifestyle brand’s main point of communication with customers is through their Instagram account, and they receive hundreds of DM’s every day. In order to create an efficient workflow for their social media managers, the company uses text analytics software to comb through DM’s and send them to the correct person or team.
Not only will the AI detect the topic, such as a specific product or subscription service, but it will also track the intent of the message, such as returns, cancellations, changing a shipping address, purely informational, etc. For example, one specific social manager may be in charge of responding to informational requests about a new product, but a customer service rep would handle any returns of the same product. Based on the detected intent, the AI will forward the DM to the correct person.
4. Data Extraction
Data extraction using AI is pretty straightforward to understand. Instead of analyzing keywords to classify a broader topic and intent, data extraction concerns only the actual information available within the text. This can be used for a wide variety of business practices such as extracting demographic information from a set of negative reviews or compiling the titles of people who sign up for a newsletter in order to build new customer personas.
Real-World Example: A major global company recently experienced a financial scandal, and are working to craft PR messaging to stakeholders. In order to get a holistic view of how much information is in the news cycle and what people are saying, they use a data extraction tool to pull links for all news articles that have headlines containing the name of the company, the names of the employees involved, the words “financial scandal,” or any other terms they deem relevant. If they wanted, they could then sort these articles further using topic and/or sentiment analysis.
5. Sentiment Tracking
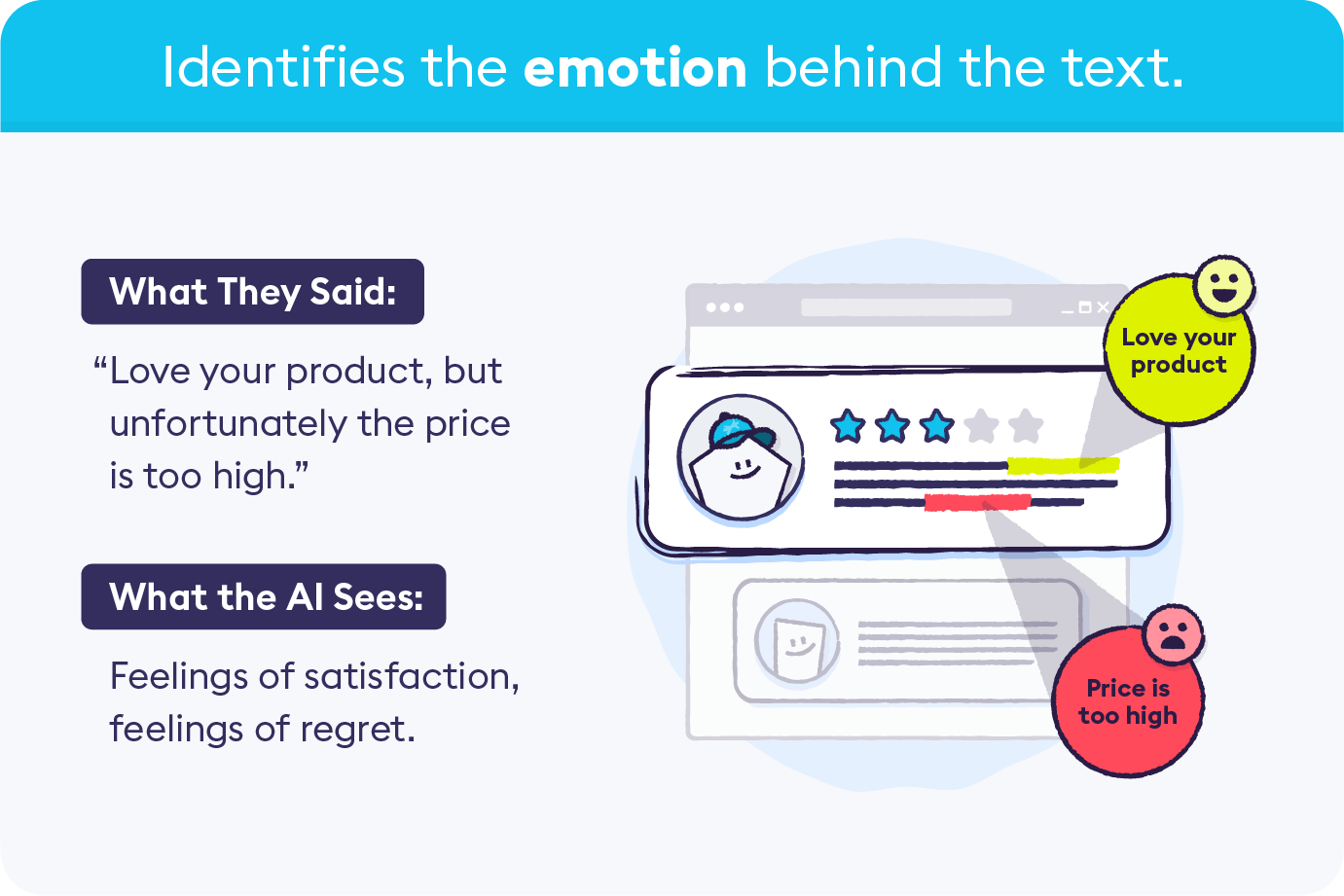
Sentiment tracking or sentiment analysis is a natural next step when it comes to extracting the most insightful data from qualitative text. Sentiment analysis is similar to topic classification, where the AI is taught to recognize certain key phrases within the text, and assigned rules for what to do with text that contains those key phrases. However, instead of analyzing the topic or intent of a piece of text, the AI analyzes the emotion or sentiment behind the words. In other words, what does the person feel about what they’re writing about?
Real-World Example: The application of sentiment tracking is obvious; you need to know how your customers are feeling about your business. For example, a company offering meal box delivery services received an email reading “I’d unfortunately like to cancel my snack box subscription. The variety is great, but the beef jerky packs are disgusting.” Using sentiment analysis, the AI will be able to classify the first part of the sentence as regretful (“unfortunately”) and the second part as highly negative (“disgusting”).
Since this company was able to compile and analyze these types of review at scale, they’ll be able to identify if this is a wider trend and work to replace the beef jerky packs, along with offering discounts to regretful customers to perhaps entice them to come back.
For a condensed version of the basics of text analytics and its business applications, take a look at the infographic below:

After reading through these examples and explanations, it’s clear that the best insights for your company will come from AI-backed text analytics that can combine these features. You need to be able to find out what your customers are talking about, their pain points, and what they want from you, quickly and at scale. Chattermill’s text analytics tools are unique in that the advanced AI can assign multiple sentiments to a single topic in a single piece of text, therefore drawing the most accurate conclusions about a piece of feedback. Contact us for a demo to try it out for yourself.